How is AI’s synthetic data enhancing User Experience Research?
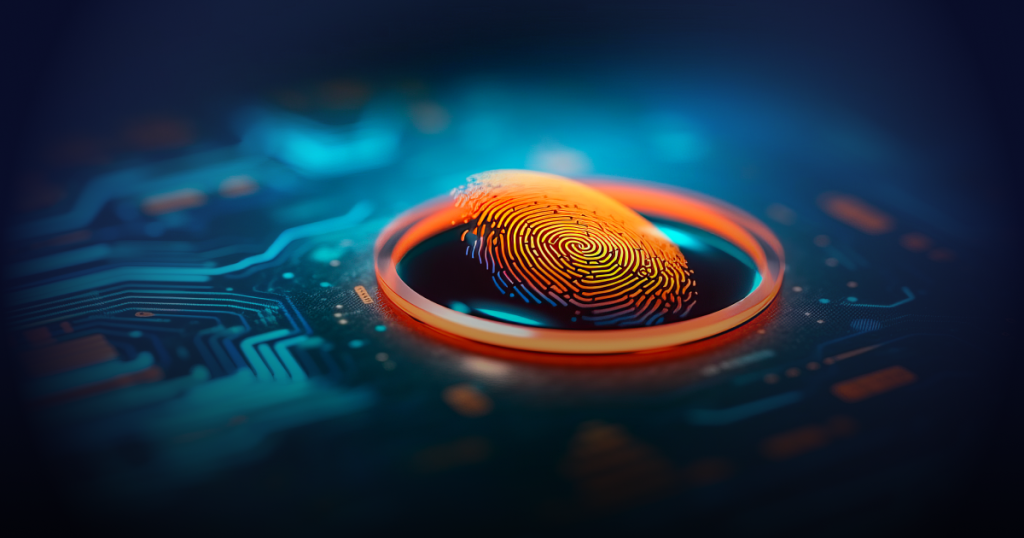
Synthetic data has found widespread application in domains such as machine learning and computer vision, yet its potential in user experience research (UXR) is gaining traction in the advancing era of artificial intelligence (AI).
Synthetic data finds versatile applications, addressing critical needs in fields such as:
- Medicine: by addressing the scarcity of annotated data in medical image processing, offering diverse training data for medical applications.
- Crowd management: where governments utilize synthetically simulated crowds to ensure crowd comfort and prevent overcrowding, using principles from physics, psychology, and human behaviour.
- Autonomous vehicle testing: by creating synthetic environments through computer graphics and physics-based modelling.
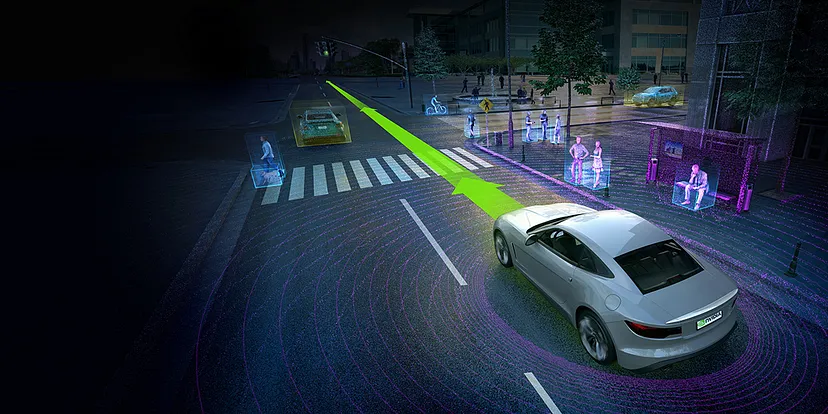
Advantages and challenges of synthetic data in UXR
UX research traditionally relies on resource-intensive methods like surveys and interviews. The rise of generative AI (GenAI) tools presents opportunities for streamlining tasks such as annotating qualitative data and crafting interview guides.
A study conducted within a luxury goods brand experimentally tried Synthetic Users, a platform for testing on AI ‘users’, alongside real-life customer interviews. While synthetic responses closely resembled human ones, they tended to lack specificity. However, they proved valuable for ideation, pilot research, and validating research questions.
Debates within the UXR community reflect nuanced perspectives. Studies demonstrate promising results of synthetic data in idea generation and pilot experiments, there are still concerns about making sure the data is accurate and used correctly.
Critiques highlight the limitations of AI tools such as ChatGPT in verifying assumptions, often ‘hallucinating’ new ones. And, the inconsistency between what users say and do underscores the complexity of capturing human experiences through AI alone.
Synthetic tools risk oversimplification, potentially overlooking uncommon cases, that actually are crucial in certain contexts like diagnosing rare conditions. Addressing this requires intentional inclusion of synthetic data on rare diseases in model training, for example.
Synthetic UXR serves as a complementary tool, enhancing efficiency in piloting studies and refining research questions. While it is great for exploring overlooked dimensions, it cannot replace the insights derived from real user data.
Looking forward
Embracing synthetic UXR prepares researchers for the AI-driven future, offering opportunities to validate outputs with real user data and enhance algorithm explainability. However, the indispensability of real user data in creating products that excel in the real world remains essential.
Do you want to know more? Read the full article from our experts at EPAM here!